Teaching AI to remember
What do people’s brains and deep neural networks have in common? They both can be forgetful.
However, human brains take advantage of a mechanism referred to as ‘replay’ to protect memories against forgetting. Inspired by this brain mechanism, researchers at Baylor College of Medicine have developed a new method to protect deep neural networks, found in artificial intelligence (AI), from forgetting what they have previously learned.
Deep neural networks are the main drivers behind the recent fast progress in AI. These networks are extremely good at learning to solve individual tasks. However, when they are trained on a new task, they typically lose the ability to solve the previously learned task completely. This phenomenon, known as “catastrophic forgetting,” is a major obstacle to the development of AI applications capable of lifelong learning.
Human helping machine
In stark contrast, humans are able to continuously accumulate information throughout their life.
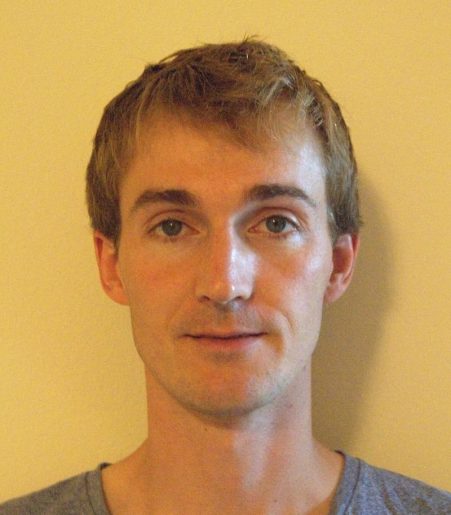
“An important mechanism in the brain thought to protect memories is the replay of neuronal activity patterns representing those memories,” said Dr. Gido van de Ven, lead author of the study and postdoctoral associate in the Department of Neuroscience at Baylor. “Such a replay mechanism had previously been used in the deep learning community (those working in AI) to alleviate catastrophic forgetting, but these earlier attempts either required storing large amounts of data, which is biologically implausible and not always possible in practice, or they could not scale to more challenging, real-world problems.”
“In our study, instead of storing data, we teach the network itself to generate representations of what it has seen before,” said Dr. Andreas Tolias, senior author of the work and director of the Center for Neuroscience and Artificial Intelligence at Baylor. “We also don’t ask the network to generate detailed, pixel-level images but rather abstract, high-level representations. We found that this kind of ‘generative replay’ is surprisingly efficient; replaying just a few of those generated representations can already be enough for the process to work.”
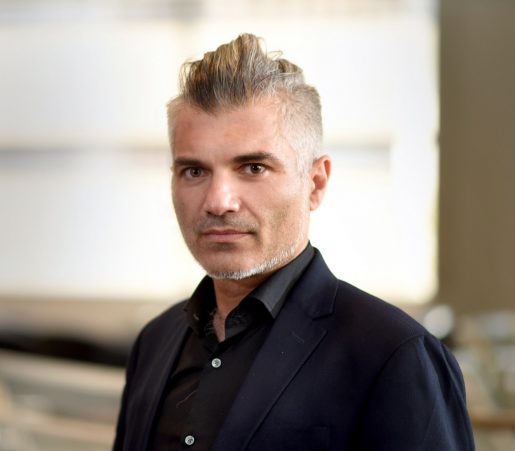
Besides using insights from neuroscience to fix shortcomings of deep learning, another goal of the study was to improve understanding of the computational role of replay in the brain.
“Our method makes several interesting predictions about the way replay might contribute to memory consolidation in the brain,” said van de Ven.
“We are already running an experiment to test some of these predictions,” added Tolias.
Read the complete article in of Nature Communications.
Another contributor to this study was Dr. Hava Siegelmann from the University of Massachusetts, Amherst.
This work was supported by an IBRO-ISN Research Fellowship, by the Lifelong Learning Machines (L2M) program of the Defence Advanced Research Projects Agency (DARPA) via contract number HR0011-18-2-0025 and by the Intelligence Advanced Research Projects Activity (IARPA) via Department of Interior/Interior Business Center (DoI/IBC) contract number D16PC00003.