Sorting out cell type-specific molecular barcodes
In the last 10 years, scientists generated thousands of whole genome bisulfite sequencing (WGBS) data sets costing millions of dollars, yet were unable to appreciate much of the information available in the data. “Now, for the first time, researchers can ‘tune in’ to the full richness and complexity of WGBS data,” said Dr. Robert A. Waterland, professor of pediatrics – nutrition at the USDA/ARS Children’s Nutrition Research Center at Baylor College of Medicine and Texas Children’s Hospital.
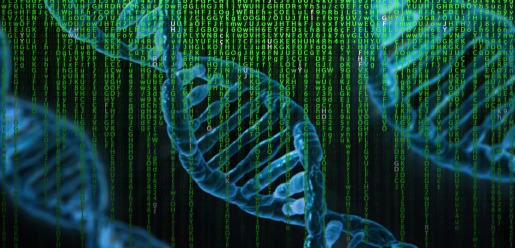
WGBS is a next-generation sequencing technology, the current gold-standard approach to determine DNA methylation of each cytosine, one of the DNA building blocks, in the entire genome. DNA methylation is one of the epigenetic mechanisms that cells use to turn genes on and off. Understanding methylation patterns can improve our understanding not only of normal development and cellular differentiation, but also disease.
“WGBS studies typically report the average methylation level at each cytosine. However, in tissues with multiple cell types, this average reflects a mashup of the methylation level of each cell type in the mixture, obscuring cell-type specific differences,” said Dr. Cristian Coarfa, associate professor of molecular and cellular biology and part of the Center for Precision Environmental Health at Baylor.
Existing analytical approaches can not distinguish methylation signals arising from those different cell types.
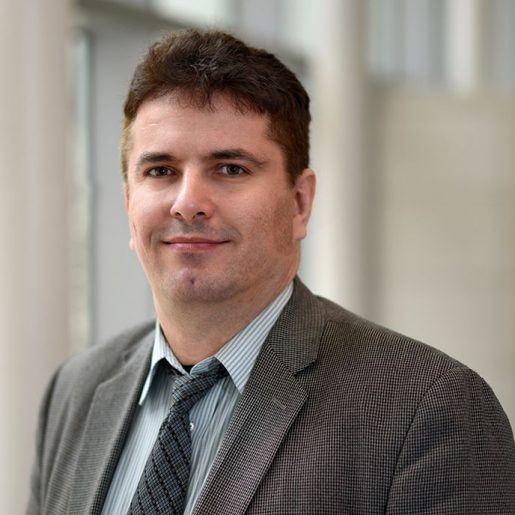
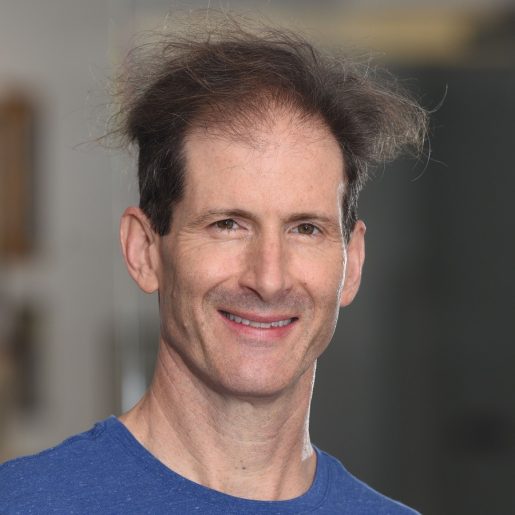
Identifying cell type-specific molecular barcodes
“The key insight that motivated the current study is that the DNA sequence ‘reads’ in WGBS data are direct descendants of DNA molecules originating from different cells of the tissue. We postulated that the methylation ‘patterns’ we detect on tissue sequencing reads contain information about what cell types the reads originated from,” said Waterland, also a professor of molecular and human genetics at Baylor. “To test this we developed software that identifies these cell type-specific methylation patterns within bulk WGBS data. This software is called Cluster-Based analysis of CpG methylation (CluBCpG).”
As one validation, the researchers used CluBCpG to analyze WGBS datasets from two types of human immune cells, B cells and monocytes. They were able to identify more than 100,000 unique molecular barcodes within each cell type. Then, they applied their method to mixtures of reads from another WGBS dataset from these two cell types, from entirely different people.
“Just by counting occurrences of these molecular barcodes in the novel datasets, CluBCpG allowed us to precisely determine the percentage of B cells and monocytes in each mixture,” said Dr. C. Anthony Scott, former postdoctoral researcher in the Waterland lab and co-first author on the paper. “We also showed that these cell-type specific signals are associated with cellular functions in different types of human and mouse brain cells and blood cells, and that they can even predict which genes are expressed.”
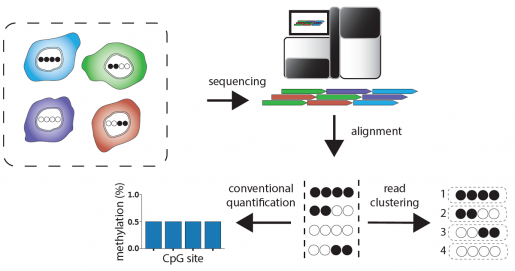
Boosting the information content of existing datasets
The CluBCpG software works together with a second development, a sophisticated machine-learning software package called Precise Read-Level Imputation of Methylation (PReLIM). This software ‘fills in’ missing information on sequencing reads that cover some of the sites in a region, increasing the information content of existing WGBS datasets by 50 to 100 percent.
“PReLIM learns from the hundreds of millions of reads in each WGBS dataset to predict the methylation state at missing sites on individual sequence reads,” said Jack D. Duryea, former student in the Waterland lab and co-first author on the paper. “We showed that PReLIM’s predictions are correct 95 percent of the time.”
Since WGBS datasets cost thousands of dollars to generate, getting 50 to 100 percent more data – at no extra charge – is a big deal.
These new computational tools, published in the journal Genome Biology and available for free download, can be applied to existing whole-genome methylation datasets from any species. This opens exciting new possibilities to improve our understanding of how DNA methylation regulates cellular function.
The researchers anticipate these new computational developments will be applied to study methylation differences in normal cells as well as in disease.
“For instance, these methods will provide better resolution in studies aiming to identify methylation differences between a healthy brain and one with a disease. We might be able to determine, for example, that epigenetic changes linked to a disease occur only in one specific type of brain cell, which would be a major step toward understanding a disease,” Waterland said.
Other contributors to this work include Harry MacKay, Maria S. Baker, Eleonora Laritsky and Chathura J. Gunasekara, all at Baylor College of Medicine.
This work was supported by NIH/NIDDK (grant numbers 1R01DK111522 and 1R01DK111831), the Cancer Prevention and Research Institute of Texas (grant number RP170295) and USDA/ARS (CRIS 3092-5-001-059).